RAG vs Fine-Tuning for Conversational AI
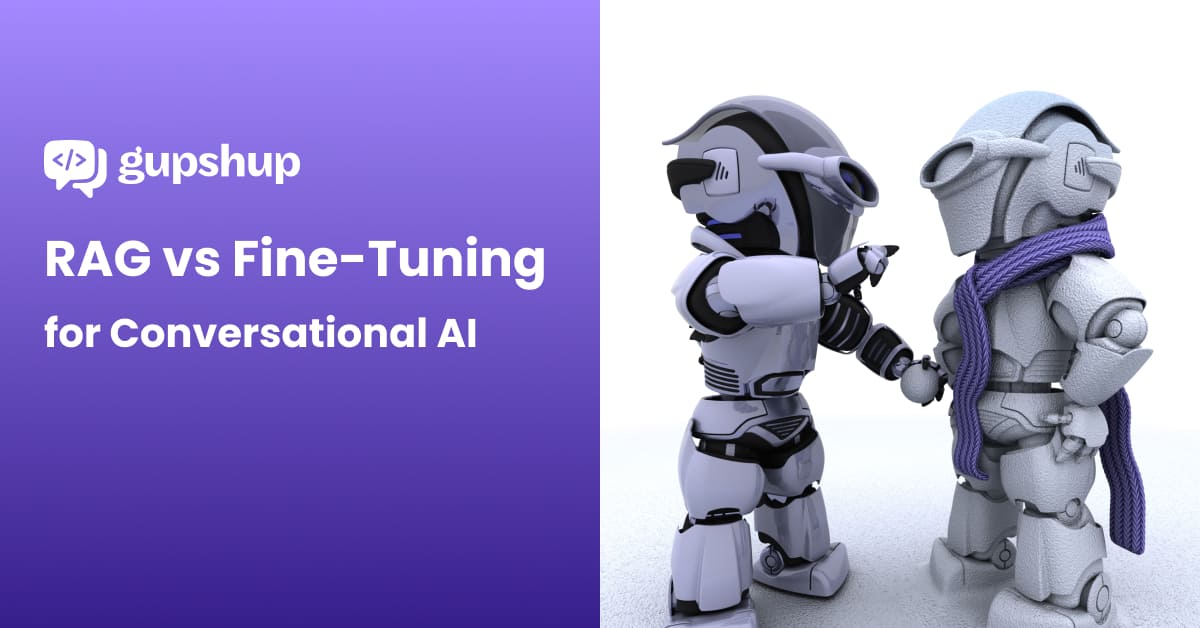
AI-driven conversations have moved from being a futuristic “nice-to-have” to an essential business advantage. Whether it’s answering customer questions, assisting sales teams, or supporting internal operations — conversational AI is reshaping how companies engage and scale.
But deploying AI agents isn’t just about picking a model and going live. Instead, it starts with a critical decision: what is the right approach for your needs?
Should you implement Retrieval-Augmented Generation (RAG), fine-tune Large Language Models (LLMs), or combine both approaches?
Both have powerful capabilities. Both offer unique benefits. But each also comes with some challenges you need to be aware of.
Choosing the right one — or knowing when to combine them — can not just shape your customer experience but also help in controlling operational costs, and driving business outcomes.
In this blog, we’ll break down RAG and Fine-Tuning in simple terms, explore real-world examples, and help you navigate which approach fits your goals best — whether you’re looking for factual accuracy, optimizing implementation costs, maintaining brand voice consistency, or balancing all three. We’ll also help you assess if your organization is truly ready to undertake the resource-intensive development efforts that fine-tuning requires, or whether leveraging existing solutions might deliver better ROI.
Understanding the Building Blocks: RAG vs Fine-Tuning Explained
RAG in Simple Terms
Retrieval-Augmented Generation (RAG) works like an AI with access to a reference library. Put simply, RAG allows LLMs to look things up before they answer. When a customer asks a question, the system:
1. LLM searches through a knowledge base (company documentation, product manuals, FAQs, help docs, internal wikis etc.)
2. Retrieves the most relevant information
3. Uses that retrieved information to generate an accurate, contextual response
Think of it as an AI agent that checks your company’s documentation before answering a customer’s question.
Real-world example: Gupshup’s Banking Relationship Manager AI Agent uses RAG to access up-to-date policy information, interest rates, and account details to provide precise answers about banking products and services.
The AI provides precise information because the knowledge base is regularly refreshed with current rates, policy changes, and promotional offers—ensuring customers always receive the most up-to-date information without requiring model retraining.
Fine-Tuning in Simple Terms
Now imagine your AI is like a personal assistant who’s worked with your team for years. You’ve trained it on all your past conversations, tone, jargon, and behavior.
Fine-Tuning means retraining a foundational LLM on specific examples — such as your brand’s customer service chat logs, documents, tone of voice, and domain language. It learns deeply and responds as if it already knows your business. It is more like teaching an LLM your business. Through additional training on your specific data, the model learns:
1. Your products and services
2. Your brand’s communication style
3. How to handle common customer scenarios
After fine-tuning, the model has internalized this knowledge and doesn’t need to look up information for every query.
When to Use What: Real-World Examples
When to Use RAG: Use Cases and Examples
1. Dynamic Content & Constantly Changing Information
Best for: Industries where product catalog, policies, pricing, inventory availability or procedures change frequently.
When your business operates in a dynamic environment with constant updates to products, services, or policies, RAG provides a significant advantage. Since the system retrieves information in real-time, you can simply update your knowledge base, and the conversational AI agent immediately has access to the latest information—no retraining required.
Example:
- An electronics retailer updates its warranty terms every quarter. A customer asks about the warranty on a newly launched model.
→ Gupshup’s RAG-powered Warranty Sales AI agent fetches the latest product sheet and gives an up-to-date, legally accurate response. - Gupshup’s Order Management AI Agent uses RAG to access the latest delivery status information from your logistics partner, enabling it to provide accurate tracking updates even as shipping details change throughout the day.
2. Factual and Policy-Based Answers
Best for: When precision and accuracy are non-negotiable
If your business needs precision in responses — like about products, account details, policies, legal disclaimers, compliance, or financial data — RAG ensures accuracy by grounding responses in reality.
Example:
- A fintech app user asks, “What are the current home loan interest rates for salaried professionals?”
→ RAG powered Gupshup’s Loan Assistance AI Agent references the latest product PDF and answers with the exact rate, eligibility, and T&Cs.
3. Complex Knowledge Bases
Best for: When documentation is extensive and detailed
Organizations with vast documentation benefit from RAG’s ability to search through and retrieve information from extensive knowledge bases. In simpler terms if you have thousands of FAQs or technical documents, RAG helps navigate and serve answers without needing to memorize everything.
Example:
Gupshup’s Healthcare AI Agents leverage RAG to navigate through 10-15K FAQs to provide accurate health advice while maintaining appropriate medical disclaimers and referring to professional care when necessary.
When to Use Fine-Tuning: Use Cases and Examples
1. Domain Expertise and Customer Journey Understanding
Best for: When deep domain knowledge and customer journey expertise are critical
Fine-tuning helps your AI agent truly understand the nuances of an industry and different stages across the customer journey funnel. Rather than simply responding to queries, a fine-tuned model can recognize where customers are in their journey and provide appropriately tailored guidance.
Gupshup’s AI uses fine-tuning to develop specialized capabilities like making contextually relevant product recommendations, understanding complex purchasing decisions, and effectively guiding customers toward completing transactions. For example, in retail banking, the AI recognizes when a customer is researching mortgage options versus when they’re ready to submit an application, adjusting its approach accordingly to provide the right level of information and assistance.
Example:
A retail banking customer interacts with a fine-tuned AI agent during their home-buying journey. Instead of simply answering: “Our mortgage rate is 5.75%,”
the fine-tuned model says:
“I see you’re exploring mortgage options. Our current rate is 5.75%, but as a first-time homebuyer, you might qualify for our special program with lower down payment requirements. Would you like me to walk you through your options based on your budget?”
2. Specialized Domain Knowledge
Best for: Industries with unique terminology and concepts
In specialized fields with domain-specific language, fine-tuning helps the AI understand industry terminology and concepts without constant reference to documentation.
Example: The industry-trained GPTs for banking, healthcare, and automotive sectors are fine-tuned on domain-specific knowledge, enabling them to understand specialized terms and processes.
For instance, fine-tuning for Banking starts with collecting and processing training data. Gupshup draws on deep domain expertise, proprietary data sources, human-created and synthesized examples, and relevant public datasets to create a high-quality dataset specifically tailored for banking applications. Data synthesis is not straight-forward either, as it takes months to ascertain what kind of data will be useful for training.
3. Predictable Query Patterns
Best for: When most questions fall into expected categories
When customer interactions follow predictable patterns, fine-tuning can prepare the AI to handle these common scenarios efficiently.
Example: An EMI Collections use case leverages a fine-tuned model such as ACE LLM to handle common payment-related queries, terms & conditions, explaining to customers implications of late payments, understanding payment difficulties and offering appropriate advice.
4. Complex Decision-Making Requirements
Best for: Scenarios requiring nuanced judgment based on implicit knowledge
In cases where decisions rely on subtle factors not explicitly documented, fine-tuning can help the model develop the judgment needed for these situations.
Example: Financial advice requires deep understanding and a fine-tuned LLM that has been trained on thousands of previous customer interactions can better understand the implicit factors that influence appropriate financial recommendations while maintaining compliance with regulations.
The Power of Hybrid Techniques
For many businesses, the most effective approach combines both RAG and fine-tuning to leverage the strengths of each method.
1. Complex Customer Journeys
Best for: Combining factual accuracy with nuanced understanding
Customer journeys that involve both specific information retrieval and nuanced understanding of customer intent benefit from a hybrid approach.
Example: Gupshup’s Property Discovery AI Agent combines RAG for retrieving specific property details with fine-tuned logic for qualifying prospects and understanding their housing preferences and constraints.
2. Multi-Function Support Systems
Best for: When the same agent handles diverse interactions
AI systems that need to perform various functions—from information provision to complex problem-solving—benefit from both approaches.
Example: Gupshup’s Travel & Hospitality AI Agent uses both approaches to handle bookings (using RAG for accurate availability and pricing) while providing personalized recommendations based on fine-tuned understanding of traveler preferences.
Use Case Matrix for RAG, Fine-Tuning, and Combination Approaches
When deciding between RAG, Fine-Tuning, or a Hybrid approach for your conversational AI, it’s important to consider the specific needs of your business. Below, we’ve mapped out various use cases to help you understand which method works best for different scenarios and industries.
Use Case
Best Fit Why Example
Constantly Changing Content (policies, SKUs, features) RAG RAG keeps information updated without needing retraining. E-commerce - pulling live data to give accurate details on new product arrivals, offers, item availability or discounts.
High-Touch Industries (finance, luxury, healthcare) Fine-Tuning Ensures brand-specific tone, empathy, and domain expertise. Healthcare AI Agent providing empathetic responses with medical knowledge about symptoms and treatments.
Multilingual Support Across Markets RAG RAG can pull information from localized documents or databases on the fly. Travel AI Agent fetching up-to-date hotel information and policies in various languages.The system also pulls real-time weather data to advise travelers on what to pack.
Need Both Freshness & Tone (e.g., FAQs and conversational support) Hybrid Combines RAG for up-to-date info and Fine-Tuning for brand-specific tone and personalized responses. Retail AI Agent providing product recommendations (RAG) and customer service with a luxury brand tone (Fine-Tuning).
Handling Highly Specialized Domain Knowledge Fine-Tuning Fine-tuning enables deeper understanding of industry-specific terminology. Legal AI Agent fine-tuned on law firm documents and case precedents to answer clients accurately with guardrails that maintain confidentiality, include appropriate disclaimers, and flag complex issues for attorney review..
Real-Time Information (stock levels, order status, etc.) RAG RAG provides real-time data retrieval from dynamic sources. Order Management AI Agent pulling live data on stock levels or delivery status for real-time updates.
Handling Multiple Functions (bookings, recommendations, customer service) Hybrid Combines RAG for accurate product details and Fine-Tuning for conversational flow and personalized service. Travel & Hospitality AI Agent booking flights, recommending destinations, and assisting with customer service inquiries.
Long-Term Knowledge Retention and Contextual Understanding Fine-Tuning Fine-tuning builds a model with memory and contextual understanding over time. Insurance Claims AI Agent studies all past claims and settlements to evaluate evidence for new claims to determine admissibility, and provides tailored support based on customers' claims history and interaction patterns..
Handling Complex, Less Frequent Queries RAG RAG ensures accuracy by fetching information for queries that don’t have pre-defined answers. Banking AI Agent fetching live, updated terms and conditions for loan eligibility or interest rates when asked by a customer.
Need for Speed (low latency in responses) Fine-Tuning Fine-tuning allows for fast responses as it doesn't rely on external queries. E-commerce AI Agent trained on product catalogs instantly answers customer questions about item details, availability, and compatibility, making the shopping experience faster and smoother.
Pros and Cons of RAG vs. Fine-Tuning
Every AI strategy comes with its own strengths and limitations. While RAG offers agility and real-time accuracy, Fine-Tuning delivers depth, consistency, and speed..
Understanding the benefits and challenges of each approach can help you make an informed decision based on cost, speed, maintenance effort, and business outcomes.
RAG (Retrieval-Augmented Generation)
Pros | Cons |
---|---|
Easily updated with new information without retraining (updates in minutes to hours) | Requires a well-organized and up-to-date knowledge base |
Direct access to the latest company documentation | May struggle with questions not covered in the documentation |
Transparent citations to source material, improving trust and compliance | Higher per-query computing costs due to real-time retrieval operations |
Reduced hallucinations when reliable information exists | Slower runtime compared to pre-trained models |
Faster deployment with minimal training requirements (initial setup: 1–4 weeks) | Ongoing costs include vector database infrastructure, content management, and knowledge base maintenance |
LLM Fine-Tuning
Pros | Cons |
---|---|
Strong understanding of domain-specific concepts and internalized knowledge | Requires full retraining when business or documentation changes (updates take weeks to months) |
Consistent tone, communication style, and brand voice | Higher upfront investment in ML engineering, compute, and infrastructure |
Handles implicit queries and edge cases with greater reasoning ability | Risk of hallucination in unfamiliar scenarios or when faced with new data |
Faster runtime as there's no retrieval step involved | Needs significant volumes of clean, relevant, curated training data |
Suitable for stable environments with less frequent updates (initial setup: 8-25 weeks) | Long-term maintenance includes retraining costs, hosting, and expert oversight, intensive computational requirements across multiple GPUs, and substantial memory storage due to the large size of the LLM. |
Benefits Summary:
Benefit Area | RAG (Retrieval-Augmented Generation) | Fine-Tuning |
---|---|---|
Accuracy | Pulls real-time info from a knowledge base, ensuring factual and traceable answers | Learns domain-specific patterns and language for consistent, intuitive responses |
Hallucination Control | Reduces risk of incorrect answers by sticking to verified content; can say "I don’t know" | Can be trained to recognize uncertainty and respond cautiously in unfamiliar situations |
Deployment Speed | Fast to launch with existing content; updates are quick—no retraining needed | Slower setup due to training needs, but offers long-term consistency once established |
Customer Experience | Best for factual, technical, or policy-related queries, even edge cases | Delivers smoother, brand-aligned conversations with better multi-turn context handling |
Real-World Use Case: How Petromin Leverages RAG to Deliver Timely Offers
Petromin’s Autocare AI Agent demonstrates Retrieval-Augmented Generation (RAG) at its finest. Developed in partnership with Gupshup, this automatically delivers only current, relevant promotions to customers based on their specific category interests.
Eliminating the need for manual offer database updates, the AI Agents scrapes offer from the website and updates in the RAG database. This ensures customers never receive expired offers, but get timely information on live promotions that match their needs.
This reduces administrative burden while significantly improving marketing accuracy and relevance.
Businesses like Petromin personalize content and offers at scale.
Decision Framework: Choosing the Right Approach
Choosing between RAG and Fine-Tuning isn’t about right vs wrong — it’s about what works best for your business stage, data availability, and customer expectations.
- RAG is ideal for most businesses — especially if your documentation is solid and updated often.
- Fine-Tuning works best for enterprise-grade needs where tone, compliance, or specialization matter.
- Hybrid delivers the gold standard — if you’re ready for complexity and want to truly scale smart conversations.
Thus, both Retrieval-Augmented Generation (RAG) and LLM Fine-Tuning bring unique strengths to conversational AI. RAG shines in environments with fast-changing content, offering real-time access to accurate information. Fine-tuning, meanwhile, excels at delivering consistent tone, deeper domain understanding, and a more natural conversation flow.
Businesses should think hard before undertaking fine-tuning independently. The effort required is substantial, demanding specialized ML expertise, significant computing resources, extensive data preparation, cleaning, and validation. The process typically takes 3-8 weeks initially, with ongoing maintenance needs. Consider whether the investment is worthwhile based on your long-term strategy, available resources, and how long your information will remain relevant before requiring updates.
However, by combining these approaches, businesses can create powerful AI agents that are not only factually reliable but also context-aware and brand-aligned. This hybrid strategy delivers the best results by combining the strengths of both methods. Start with RAG for quick deployment and factual accuracy, then strategically add fine-tuning elements for improved conversational quality in high-value, stable interaction patterns. This phased approach allows for immediate value while building toward more sophisticated experiences over time..
At Gupshup, we’ve seen firsthand how this layered, multi-modal approach—combining text, voice, and visual interfaces— approach transforms customer experiences across industries like banking, retail, healthcare, and more. Our AI agents are built to scale with your needs—fast, smart, and tailored to your brand-while leveraging a flexible LLM stack. As models become lighter and more specialized, using fine-tuned, use case–specific LLMs can meaningfully reduce costs and development effort
Ready to see it in action?
Book a consultation to explore how Gupshup’s AI Agents can elevate your customer engagement strategy.